Gartner recently released, “Infographic: Understand Intelligent Document Processing”. According to Gartner, “The market for document capture, extraction, and processing is highly fragmented. Data and analytics leaders should use this research to understand the process flow and differentiated capabilities offered by intelligent document processing solutions.” In this series of posts, we speak to the 6 critical flows in Intelligent Document Processing (IDP) that Gartner covers and how Infrrd solutions stack up.
1. Capture or Ingestion
2. Document Preprocessing
3. Document Classification
4. Data Extraction
5. Validation and Feedback Loop
6. Integration
In this third post, we explore Data Extraction. (Check out our earlier posts in this series, Capture and Preprocessing and Document Classification.)
Why did the world need a new data extraction solution?
When people hear data extraction, the first thing that usually comes to mind is OCR. For the last several years, traditional OCR solutions have been the preferred choice for extracting data. However, OCR solutions have their share of challenges because they are primarily focused on converting handwritten or printed text into a machine-readable, digital data format.
Mere data extraction without intelligence for understanding what that data indicates is a huge waste of potential. With changing technology, businesses are benefiting from the advent of neural networks and algorithms for natural language processing or computer vision used in modern IDP solutions.
Here is a comparison table between traditional OCR and modern IDP systems:

Multiple AI technologies, such as natural language processing (NLP), computer vision, and predictive analytics, are rolled into IDP. Machine learning and AI capabilities have grown leaps and bounds and plugged the gaps OCR was not designed to address. Modern IDP solutions have an ML-first approach coupled with advanced AI technologies to seamlessly extract and organize relevant and meaningful information with high accuracy from any raw data, be it unstructured, semi-structured, or structured.
Modern IDP systems offer Intelligent Data Extraction and can handle millions of variations of documents, including invoices, receipts, loan documents, and insurance documents, without creating templates. IDP leaders, such as Infrrd, are deeply invested and focused on Intelligent Data Extraction.
In the past, businesses relied on human resources and expertise. Today, the corporate world relies on data analytics for gaining better business insights, which means Intelligent Data Extraction automatically becomes a key factor for a business.
What does IDP offer?
An efficient IDP solution addresses several data extraction challenges. The key challenge is extracting meaningful data from different types of information as follows:
1. Textual data extraction
- a. Key value pairs
- b. Entity recognition
- c. Questions and answers
2. Visual data extraction
- a. Tables
- b. Checkboxes
- c. Logos
- d. Signatures
- e. Graphs and charts
IDP can extract high-value data for your business from both textual and visual elements in the document. This is a big differentiator between OCR and IDP systems. OCRs are not designed to handle visual elements but IDP systems are built from the ground up with the goal of handling both types of content. Infrrd’s platform leverages machine learning, deep learning, computer vision, and NLP to extract data from both these content types.
Let’s next discuss how data is extracted for these content types.
Textual Data Extraction
Textual data in a document is handled using entity extraction models, a machine learning approach for detecting different entities in a document based on thousands of other documents that the system has seen in the past. These models identify and segregate a set of information based on similar or common semantic parameters. Entity extraction uses a combination of underlying methods and technologies, ranging from visual layout understanding to deep neural networks. An efficient or trained IDP system can provide high-level accuracy which can challenge the levels of human performance.
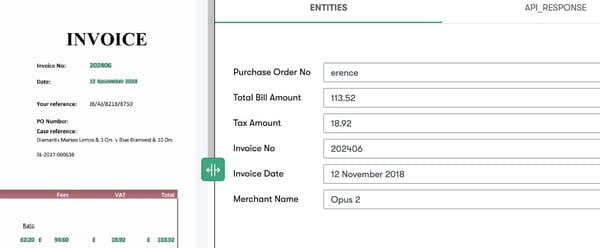
Entity extraction is the key to ensuring that you have a template-free solution that works across a wide range of documents.
Visual Element Recognition
IDP solutions are also adept at understanding visual elements such as tables, checkboxes, logos, and signatures. Extracting information from visual elements is quite complex and presents a few challenges such as:
- Denoising irrelevant content
- Detecting the region of the visual element presence accurately
- Detecting elements with multiple structures, layouts, and mostly different variations
- Detecting the exact boundaries
- Detecting sub elements in the region of interest and extracting information from them, such as rows and columns for tables
- Segmentation based on semantics
- Decoding the structural relationship of the information
Infrrd’s IDP solution already has a state-of-the-art visual element extraction feature that uses AI-based technologies, such as deep learning, neural networks, and computer vision to train machine learning algorithms. Visual element detection and extraction are beneficial only when they are accurate, effective, and cognitive in nature while handling multiple, diverse variations.
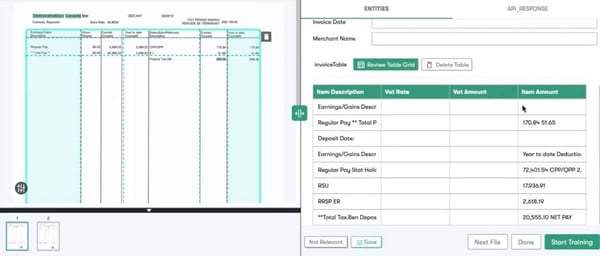
Reference: Visual Element Detection: Tables


Reference: Visual Element Detection: Checkboxes
Choosing an IDP platform that can handle both text and visual elements is critical to make sure your team does not have to open up documents for verification. It also increases your Straight-Through Processing ratio.
In our next post, we explore Gartner’s description of Validation and Feedback Loop and how Infrrd stacks up.
FAQs
Using AI for pre-fund QC audits offers the advantage of quickly verifying that loans meet all regulatory and internal guidelines without any errors. AI enhances accuracy, reduces the risk of errors or fraud, reduces the audit time by half, and streamlines the review process, ensuring compliance before disbursing funds.
Choose software that offers advanced automation technology for efficient audits, strong compliance features, customizable audit trails, and real-time reporting. Ensure it integrates well with your existing systems and offers scalability, reliable customer support, and positive user reviews.
Audit Quality Control (QC) is crucial for mortgage companies to ensure regulatory compliance, reduce risks, and maintain investor confidence. It helps identify and correct errors, fraud, or discrepancies, preventing legal issues and defaults. QC also boosts operational efficiency by uncovering inefficiencies and enhancing overall loan quality.
Mortgage review/audit QC software is a collective term for tools designed to automate and streamline the process of evaluating loans. It helps financial institutions assess the quality, compliance, and risk of loans by analyzing loan data, documents, and borrower information. This software ensures that loans meet regulatory standards, reduces the risk of errors, and speeds up the review process, making it more efficient and accurate.
IDP (Intelligent Document Processing) enhances audit QC by automatically extracting and analyzing data from loan files and documents, ensuring accuracy, compliance, and quality. It streamlines the review process, reduces errors, and ensures that all documentation meets regulatory standards and company policies, making audits more efficient and reliable.
Yes, AI can identify and extract changes in revised engineering drawings, tracking modifications to ensure accurate updates across all documentation.