Over the past 15 years, I have had the chance to work with many OCR tools and one thing I can say with certainty is that the text extraction quality of these tools has steadily improved with ongoing improvements in artificial intelligence and machine learning OCR techniques.
More than ever businesses are trying to derive useful insights and meaning from scanned images and documents. For example, banks are wanting to extract intelligence such as parties involved and contract expiry dates from scanned contracts, insurance companies are wanting to detect fraudulent receipts submitted during the claims process and many more. Use cases like these require unstructured text be converted into structured meaningful data during OCR or post OCR.
OCR tools inherently lack the intelligence to parse or understand extracted text beyond just extracting it. To assign meaning and structure to the content, another system needs to process the extracted text and extract entities and entity types from it.
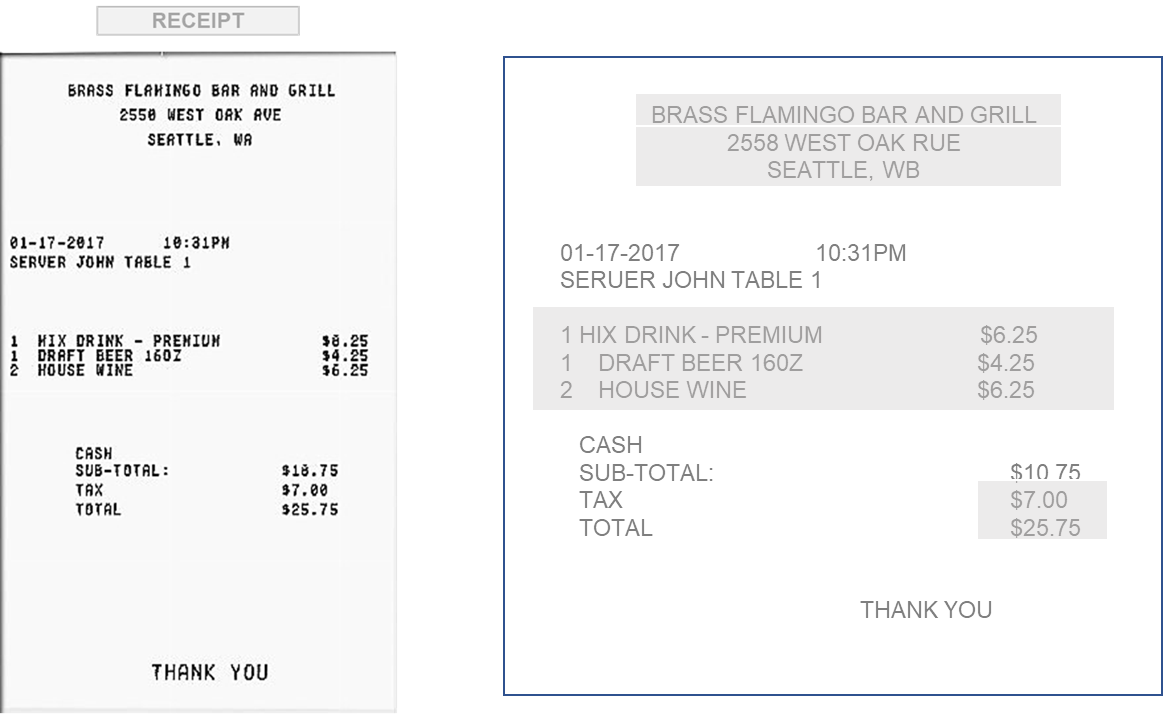
In this example, the OCR system does an accurate extraction of text however it does not have the intelligence to identify the specifics of the merchant name, merchant address or other important details such as tax, total and individual line items.
In this article, I want to compare two post-OCR text enrichment techniques. One is the conventional technique of using templates while other is the modern approach of applying machine learning.
Let’s dive into templates first. Templates are what the name says, templates. In this method, the user manually marks co-ordinates for the text of interest on the image and subsequently uses the output of OCR engine to locate and extract text. This approach works well and is highly accurate if the text layout within the scanned image matches the layout coded in the template.

However, this approach starts to fail for systems which deal with a large number of document layouts and for systems which frequently encounter new types of documents. An invoice processing system which receives new types of invoices from different suppliers is a good example. For an invoice processing system, a template approach may work fine initially but will soon become unmanageable as the number of suppliers grows and change.
Now let’s consider the alternative machine learning approach. A machine learning OCR uses a trained model which encodes thousands of rules for determining the meaning of the content. This model is generally trained using a combination of supervised and unsupervised learning methods. For example, one approach for training could be to use feature data set as follows to predict if a line in the text contains a merchant name.
A trained model can fine tune itself as more training data is collected and ingested into the training process. Machine learning approach is much more scalable across languages and across different types of documents even if they are not processed by the system. Although this approach requires that initial effort to build high-quality training models and entity recognition models, but once built, this approach scales faster and better than the templates approach.
At Infrrd we are researching and experimenting with various techniques involving machine learning to improve content enrichment post-OCR text extraction from different document types such as receipts, invoices, contracts and shipping labels.
FAQs
Using AI for pre-fund QC audits offers the advantage of quickly verifying that loans meet all regulatory and internal guidelines without any errors. AI enhances accuracy, reduces the risk of errors or fraud, reduces the audit time by half, and streamlines the review process, ensuring compliance before disbursing funds.
Choose software that offers advanced automation technology for efficient audits, strong compliance features, customizable audit trails, and real-time reporting. Ensure it integrates well with your existing systems and offers scalability, reliable customer support, and positive user reviews.
Audit Quality Control (QC) is crucial for mortgage companies to ensure regulatory compliance, reduce risks, and maintain investor confidence. It helps identify and correct errors, fraud, or discrepancies, preventing legal issues and defaults. QC also boosts operational efficiency by uncovering inefficiencies and enhancing overall loan quality.
Mortgage review/audit QC software is a collective term for tools designed to automate and streamline the process of evaluating loans. It helps financial institutions assess the quality, compliance, and risk of loans by analyzing loan data, documents, and borrower information. This software ensures that loans meet regulatory standards, reduces the risk of errors, and speeds up the review process, making it more efficient and accurate.
IDP (Intelligent Document Processing) enhances audit QC by automatically extracting and analyzing data from loan files and documents, ensuring accuracy, compliance, and quality. It streamlines the review process, reduces errors, and ensures that all documentation meets regulatory standards and company policies, making audits more efficient and reliable.
Yes, AI can identify and extract changes in revised engineering drawings, tracking modifications to ensure accurate updates across all documentation.