Forbes had predicted that 2024 would be the year of AI practicality, and as the year comes to an end, it’s safe to say they were right. What started as a modest $0.5 billion global generative AI market in 2019 has skyrocketed to an incredible $25 billion by 2024. (Source: The AI Surge of 2024)
AI agents are on the rise, finding applications in both horizontal and vertical domains. OpenAI CEO Sam Altman has called agents “AI’s killer function,” and I can’t agree more. But what functions are we talking about exactly? This is where things often get confusing. Many refer to agents when they’re actually describing the functions of assistants, and the growing integration of machine learning models into everything is further blurring the line between AI agents and AI assistants.
If you’re here looking for some clarity on this distinction, you’re in the right place. Let’s break it down.
What are AI Assistants?
If you’ve ever worked with an intern, you know you can’t just hand over a task and walk away. You have to explain the plan, give them the right tools they need, and guide them through the whole process. They might write the report, set up the meeting, create the presentation, or do whatever tasks they’re asked to do, but they rely on your direction to get it done right.
Now, this is how AI assistants work; they are efficient, task-oriented helpers that extend your instructions. When faced with a challenge, it comes back to you for help. Think of Google Assistant, Siri, or Alexa: they handle certain tasks but require constant input and are limited to straightforward activities.
In addition to persona models like AI assistants, traditional automation tools also work in a similar fashion. Take robotic process automation (RPA) software for example. RPA is often used in accounts payable (AP) to streamline tasks like vendor onboarding, processing payment schedules, and reconciling payments with ledger entries. But for this, you need to explicitly program every step: set up workflows for validating vendor information, define rules for categorizing expenses, and establish triggers for payment approvals. The tool executes exactly as instructed but cannot adapt to unexpected scenarios or make decisions outside the predefined parameters.
So, the problem here with AI assistants and similar tools is that they still depend on skilled developers to implement and maintain new features, increasing operational complexity. Incremental improvements often deliver limited efficiency gains, falling short of significant transformation.
AI Assistants Use-Cases
AI assistants are best at performing simple and straightforward small tasks, such as pulling out certain information, running periodic checks, or in just simple chats or conversations.
How Assistants Work?
Natural Language Processing (NLP) is the foundation of AI assistants, enabling them to understand queries and respond in a conversational way. Early AI assistants used rule-based systems with preprogrammed responses. Modern assistants rely on advanced machine learning and foundation models.
AI assistants use supervised learning or reinforcement learning with human feedback (RLHF) to generate responses or complete specific tasks. They often operate in a stateless manner, ie, they do not retain information about the user’s previous interactions. Take for example, when an AI chatbot times out, the conversation doesn’t resume from where you left off—you have to start over (frustrating, right?). Hence, they are limited to handling specific, prompt-driven tasks.
What are AI Agents?
Now, think of a more experienced person or your fellow team managers. When given the same task, they approach it entirely differently. They define the problem, identify key stakeholders, strategize independently, and handle the project from start to finish, keeping the larger organizational goals in mind. Even if they get stuck anywhere in case of any difficulties, they have the knowledge and experience to find a solution for it. That’s your AI agent—a goal-driven, autonomous problem-solver capable of handling problems and finding solutions whenever the need arises. AI Agents can do this because they are trained in industry-specific knowledge and have the built-in expertise to solve a problem and achieve results with foresight.
This applies to all high-end AI systems and not explicitly to persona-based AI agents.
AI Agent Use-Cases
Because AI agents excel at strategy, autonomy, and learning, they are better suited for roles requiring high-level reasoning and support. For example, you can ask an AI agent to visit a specific website, navigate through its pages to locate a particular dataset—let’s take inventory details, for example —and extract that information. The agent can then automatically open an Excel sheet, find the relevant rows or columns, and cross-check the extracted data for discrepancies or updates. If any mismatches are found, it can flag them, suggest corrections, or even update the Excel file autonomously based on predefined rules.
Therefore, as a researcher, an AI agent can gather external information from websites or databases, provide summaries, generate insights, or perform fact-checking. It uses internal instructions and feedback from external conditions to decide how to proceed and determine the most effective ways to carry out its tasks. The key distinguisher here is that agents perform high-end tasks independently with the least direct instruction.
How Do AI Agents Work?
Like AI assistants, Large language models (LLMs) form the foundation of AI agents. Responses from AI assistants are generated based on prompts, with limited planning and reasoning capabilities. In contrast, AI agents differ significantly. Their key features include tracking what has happened so far, progressing toward objectives, dynamically updating plans, and adapting to new information or environmental changes, enabling better decision-making. AI agents solve complex problems by utilizing and understanding data within the correct context and employing advanced decision-making. AI agents are applied across diverse scenarios for independent and adaptive task execution.
But the problem is, it's not as easy as it sounds. AI agents face a lot of challenges when it comes to real-life use cases. Agents demand extensive training on millions of real-world, domain-specific data points to reach a level where they can autonomously handle end-to-end tasks. This is particularly vital for them to handle complex tasks autonomously.
As a result, most agentic platforms are limited to simple automation agents that provide minor efficiency gains. However, these platforms are not designed to reduce workforce needs or manage complex workflows significantly. Engineers must invest time to learn and adapt to these tools, which often demand unique interfaces for each process, limiting their scalability and effectiveness for intricate automation tasks.
The Differentiator: Infrrd’s AI Agents Solution
Infrrd deep agents are automated agentic platforms capable of performing tasks autonomously from start to finish with better than human speed and accuracy. They are extensively trained in all major data industries like mortgage, insurance, invoice, accounting, manufacturing, and construction.
With over a decade of automation expertise in the above-mentioned industries, our agentic platform creates agents for any specific task or purpose. We train our agents with extensive data and rigorous processes to ensure they deliver the highest level of performance. They intuitively know when to escalate issues for review and when to take corrective action independently. Through comprehensive training and adaptive learning, our solution ensures exceptional accuracy and compliance while dramatically reducing turnaround times.
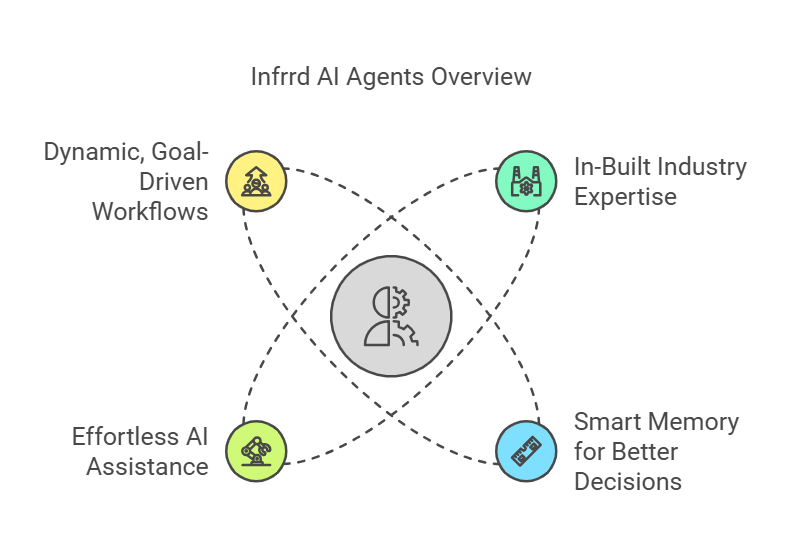
FAQs
A pre-fund QC checklist is helpful because it ensures that a mortgage loan meets all regulatory and internal requirements before funding. Catching errors, inconsistencies, or compliance issues early reduces the risk of loan defects, fraud, and potential legal problems. This proactive approach enhances loan quality, minimizes costly delays, and improves investor confidence.
A pre-fund QC checklist is a set of guidelines and criteria used to review and verify the accuracy, compliance, and completeness of a mortgage loan before funds are disbursed. It ensures that the loan meets regulatory requirements and internal standards, reducing the risk of errors and fraud.
Using AI for pre-fund QC audits offers the advantage of quickly verifying that loans meet all regulatory and internal guidelines without any errors. AI enhances accuracy, reduces the risk of errors or fraud, reduces the audit time by half, and streamlines the review process, ensuring compliance before disbursing funds.
Choose software that offers advanced automation technology for efficient audits, strong compliance features, customizable audit trails, and real-time reporting. Ensure it integrates well with your existing systems and offers scalability, reliable customer support, and positive user reviews.
Audit Quality Control (QC) is crucial for mortgage companies to ensure regulatory compliance, reduce risks, and maintain investor confidence. It helps identify and correct errors, fraud, or discrepancies, preventing legal issues and defaults. QC also boosts operational efficiency by uncovering inefficiencies and enhancing overall loan quality.
Mortgage review/audit QC software is a collective term for tools designed to automate and streamline the process of evaluating loans. It helps financial institutions assess the quality, compliance, and risk of loans by analyzing loan data, documents, and borrower information. This software ensures that loans meet regulatory standards, reduces the risk of errors, and speeds up the review process, making it more efficient and accurate.